Fine-tuning
Train XMO-Time model according to your business needs, easily achieve one-stop, one-model solution.
Training or fine-tuning a time series prediction model involves further adjustments on the basic time series model we provide, which can improve the prediction accuracy of the model in specific application scenarios.
How to Fine-tune the Model?
1. Click on create
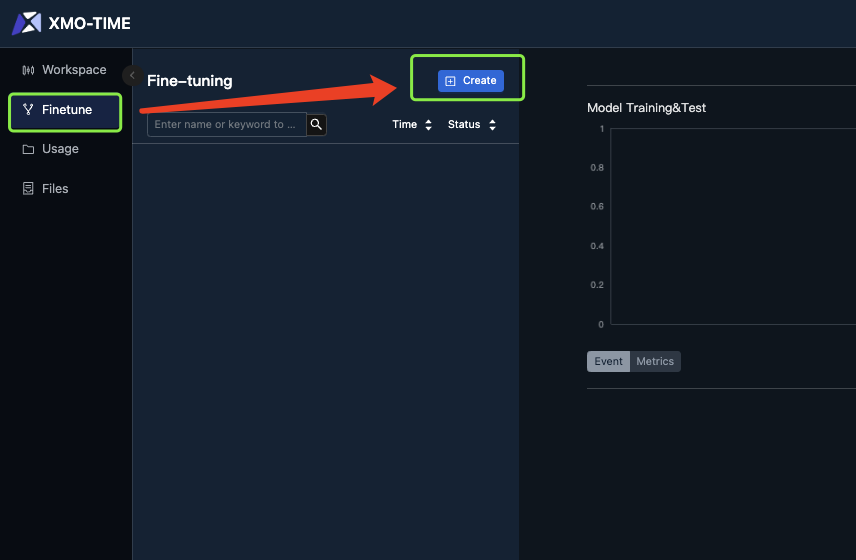
2. Choose the Base model
Select the appropriate Base model, the model provided is XMO-Time 1.0. XMO-Time is a general time series prediction model that has been extensively trained on power data, and can be fine-tuned for the power or generation of a specific photovoltaic power station.
3. Upload Training Data/Validation Data
-
- You need to prepare historical photovoltaic power data in advance.
- Split the historical data into training and validation datasets.
-
- Training Dataset
- To ensure the training effectiveness of the model, it is recommended to upload data of 3 years or more at once, or at least for 1 month, and ensure that the data is continuous and uninterrupted.
- Enter the time in the format YYYY-MM-DD hh:mm:ss; time intervals can be 5 minutes/15 minutes/1 hour/1 day per data point.
- Enter the power, unit: megawatts (MW) or kilowatts (kW), choose one.
- Specify the "Power Unit" in the "Station Information" below.
- You can optionally enter the station's longitude, latitude, installed capacity, and power unit information as needed.
-
- Validation Dataset
- The length of time can be arbitrary, ensuring that the data is continuous and uninterrupted.
- Enter the time in the format YYYY-MM-DD hh:mm:ss; time intervals can be 5 minutes/15 minutes/1 hour/1 day per data point.
- Enter the power, unit: megawatts (MW) or kilowatts (kW), choose one. Specify the "Power Unit" in the "Station Information" below.
- Optionally enter the station's longitude, latitude, installed capacity, and power unit information as needed.
4. Train the Model
After uploading the data, you need to confirm the information again. Once the information is correct, click on Confirm and Start Training. The system will fine-tune the model on the target dataset. The model training process takes approximately 60 seconds, please be patient. During the training process, network delays, data errors, and other issues may cause the model training to fail. You can click on Retry to train again, or click on Start a New Training to upload files for training again.
5. Evaluate the Model
You can view the training error and validation error on the website. Common evaluation metrics like RMSE (Root Mean Square Error) are used to validate the model and evaluate its performance on the test dataset.
6.Accuracy of Distributed Solar Power Forcast
Below is a comparison of the results of our base model XMO-time 1.0 and the fine-tuned model for the same photovoltaic power station. You can see that the fine-tuned model overall performs better than the base model.
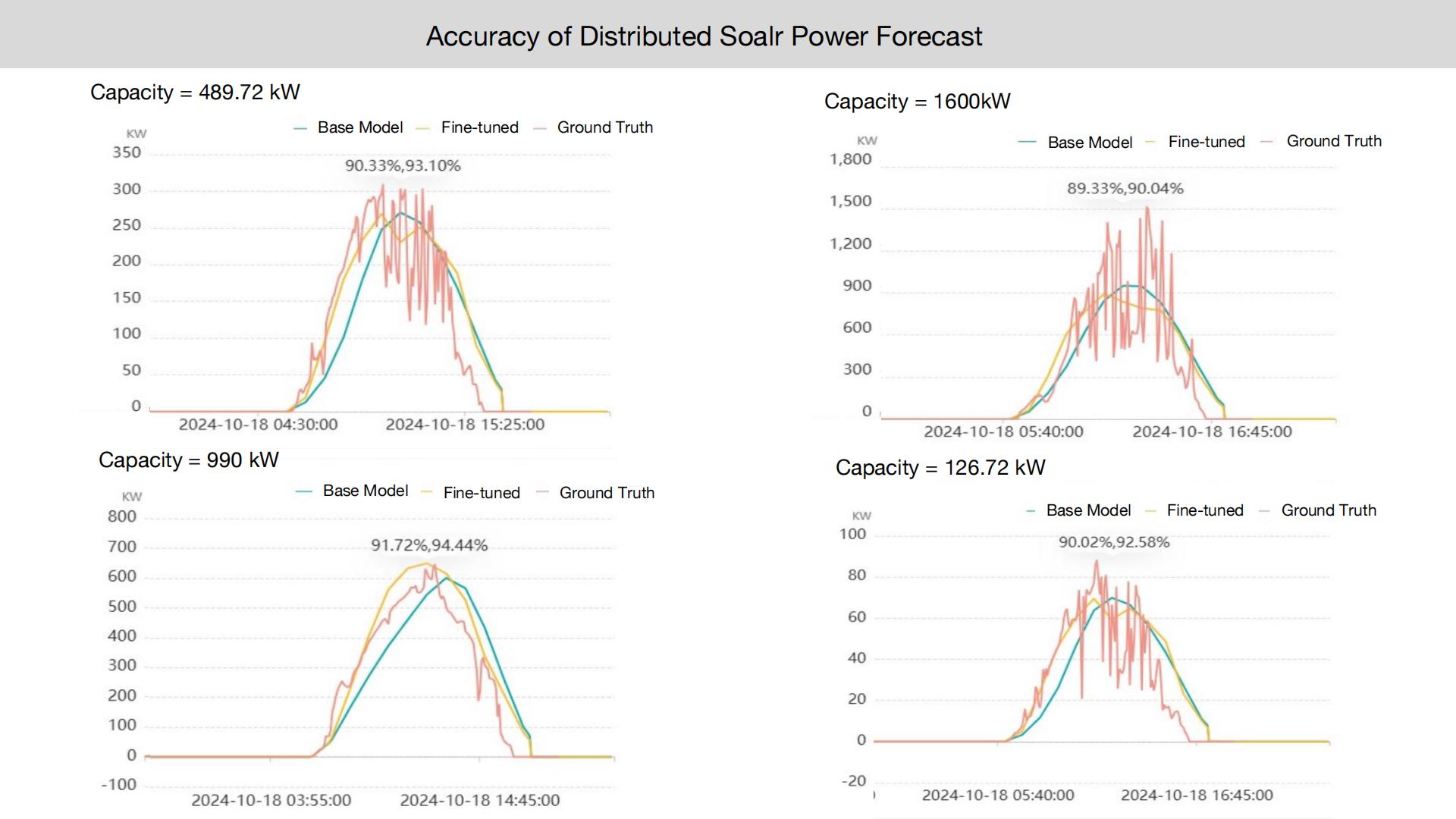